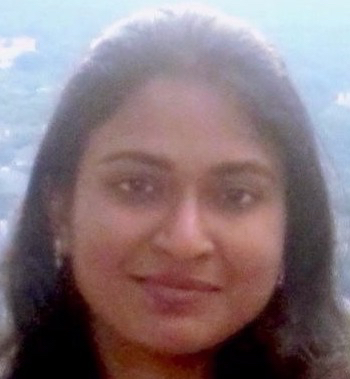
Game theoretic methods for antibody design
Abstract
Infectious diseases pose an increasing threat due to a combination of globalization, poor treatment management, and evolutionary treatment escape (that is, mutations that escape drug therapies or antibodies elicited by vaccines). The goal of this proposal is to develop highly scalable methods for sequence-based design of antibodies for HIV that exhibit very broad recognition of diverse isolates, leveraging a combination of game theoretic modeling, computational structure design, scalable sequence search, and scalable bi-level combinatorial optimization. The computational design in the combinatorial antibody and virus sequence space could create a new paradigm for developing new biologics by enabling the design of antibodies with increased breadth, high potency, and high similarity to antibodies in the human repertoire.
Description
We propose a new computational approach BROAD (BReadth Optimization for Antibody Design) that combines traditional structure-based modeling using the Rosetta software suite with machine learning and integer linear programming to overcome limitations in the Rosetta sampling methods. We use this novel method to increase predicted breadth of naturally-occurring antibody VRC23 against a panel of 180 divergent HIV viral strains and achieve 100% predicted binding against the panel. In addition, we compare the performance of this method to state-of-the-art multistate design in Rosetta and show that we can outperform the existing method significantly.
.
While broadly binding antibodies are effective, many viruses, including HIV, can rapidly escape the therapeutic effect through a sequence of mutations. Stackelberg game models have recently seen considerable practical and academic success in security applications, with defender as the leader, and attacker the follower. The key conceptual insight of Stackelberg security games is that defense needs to be proactive, optimally accounting for attacker’s response to a defensive posture. We propose that this insight has relevance in antibody design, to make virus evasion difficult. Formally, we model the interaction between an antibody and a virus as a Stackelberg game in which the vaccine designer chooses an antibody, and the virus chooses a minimal sequence of mutations to escape it. The game-theoretically designed antibody is robust to the escaping virus mutations in addition to broad binding capabilities.
Link to paper: http://vorobeychik.com/2015/abdesign.pdf
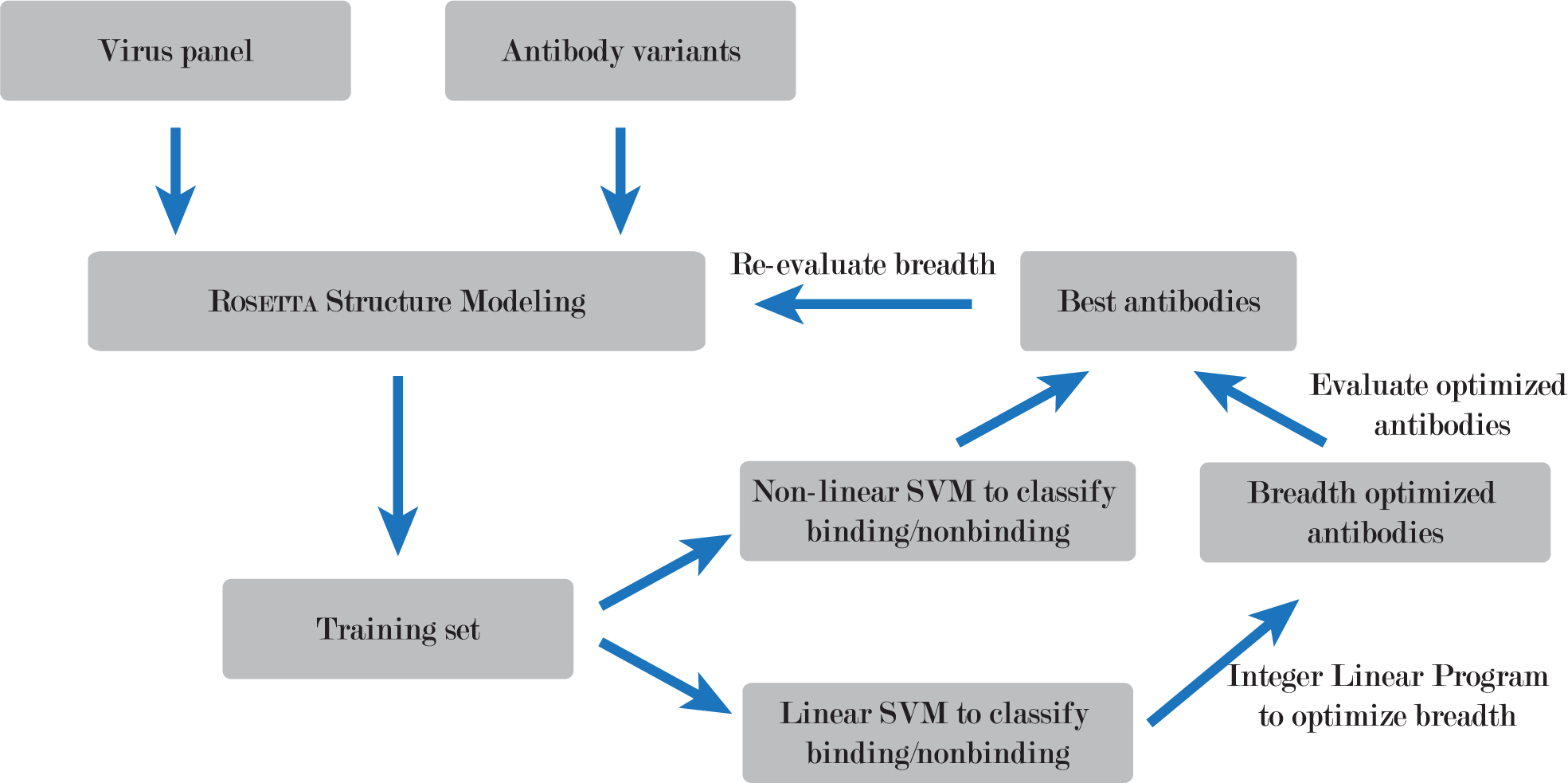
Workflow of the BROAD design method. The method uses ROSETTA structural modeling to generate a large set of mutated antibodies, support vector machines (SVM) to predict ROSETTA energy from amino acid sequence, and integer linear programming to optimize breadth of binding.
Mentors
Primary: Yevgeniy Vorobeychik
Secondary: Jens Meiler
Type of Trainee
Graduate student
©2024 Vanderbilt University ·
Site Development: University Web Communications