Robust Optic Nerve Segmentation on Clinically Acquired CT
Robert Harrigan, Swetasudha Panda, Andrew J. Asman, Michael P. DeLisi, Benjamin C. W. Yvernault, Seth A. Smith, Robert L. Galloway, Louise A. Mawn, Bennett A. Landman “Robust Optic Nerve Segmentation on Clinically Acquired CT.” Journal of Medical Imaging. 1(3), 034006 (Dec 17, 2014). † PMC3903299
Full Text: https://www.ncbi.nlm.nih.gov/pubmed/26158064
Abstract
The optic nerve (ON) plays a critical role in many devastating pathological conditions. Segmentation of the ON has the ability to provide understanding of anatomical development and progression of diseases of the ON. Recently, methods have been proposed to segment the ON but progress toward full automation has been limited. We optimize registration and fusion methods for a new multi-atlas framework for automated segmentation of the ONs, eye globes, and muscles on clinically acquired computed tomography (CT) data. Briefly, the multi-atlas approach consists of determining a region of interest within each scan using affine registration, followed by nonrigid registration on reduced field of view atlases, and performing statistical fusion on the results. We evaluate the robustness of the approach by segmenting the ON structure in 501 clinically acquired CT scan volumes obtained from 183 subjects from a thyroid eye disease patient population. A subset of 30 scan volumes was manually labeled to assess accuracy and guide method choice. Of the 18 compared methods, the ANTS Symmetric Normalization registration and nonlocal spatial simultaneous truth and performance level estimation statistical fusion resulted in the best overall performance, resulting in a median Dice similarity coefficient of 0.77, which is comparable with inter-rater (human) reproducibility at 0.73.
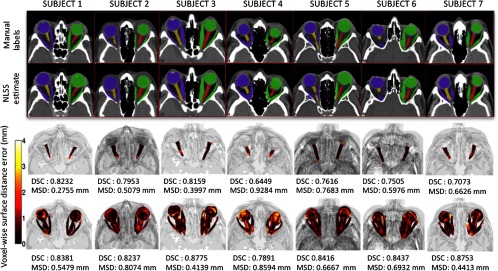