SynSeg-Net: Synthetic Segmentation Without Target Modality Ground Truth
- Yuankai Huo, Zhoubing Xu, Hyeonsoo Moon, Shunxing Bao, Albert Assad, Tamara K. Moyo, Michael R. Savona, Richard G. Abramson, and Bennett A. Landman. “SynSeg-Net: Synthetic Segmentation Without Target Modality Ground Truth.” IEEE transactions on medical imaging (2018).
Abstract
A key limitation of deep convolutional neural networks (DCNN) based image segmentation methods is the lack of generalizability. Manually traced training images are typically required when segmenting organs in a new imaging modality or from distinct disease cohort. The manual efforts can be alleviated if the manually traced images in one imaging modality (e.g., MRI) are able to train a segmentation network for another imaging modality (e.g., CT). In this paper, we propose an end-to-end synthetic segmentation network (SynSeg-Net) to train a segmentation network for a target imaging modality without having manual labels. SynSeg-Net is trained by using (1) unpaired intensity images from source and target modalities, and (2) manual labels only from source modality. SynSeg-Net is enabled by the recent advances of cycle generative adversarial networks (CycleGAN) and DCNN. We evaluate the performance of the SynSeg-Net on two experiments: (1) MRI to CT splenomegaly synthetic segmentation for abdominal images, and (2) CT to MRI total intracranial volume synthetic segmentation (TICV) for brain images. The proposed end-to-end approach achieved superior performance to two stage methods. Moreover, the SynSeg-Net achieved comparable performance to the traditional segmentation network using target modality labels in certain scenarios. The source code of SynSeg-Net is publicly available (https://github.com/MASILab/SynSeg-Net).
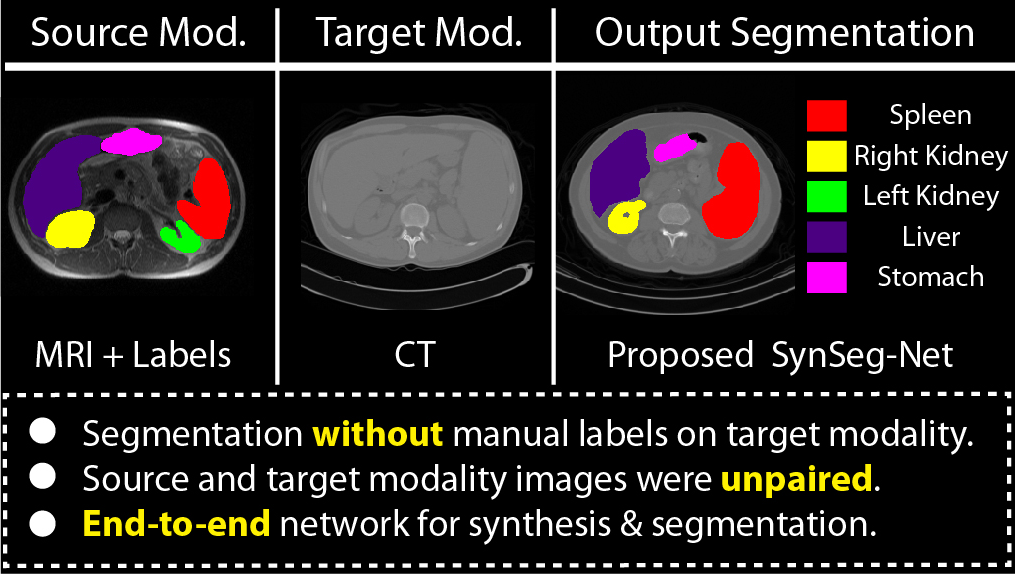