Internal-transfer Weighting of Multi-task Learning for Lung Cancer Detection
Yiyuan Yang, Riqiang Gao*, Yucheng Tang, Sanja L. Antic, Steve Deppen, Yuankai Huo, Kim L. Sandler, Pierre P. Massion, Bennett A. Landman, “Internal-transfer Weighting of Multi-task Learning for Lung Cancer Detection,” SPIE MI:IP 2020. Houston, TX.
[full text]
Abstract
Deep learning has achieved many successes in medical imaging, including lung nodule segmentation and lung cancer prediction on computed tomography (CT). Recently, multi-task networks have shown to both offer additional estimation capabilities, and, perhaps more importantly, increased performance over single-task networks on a “main/primary” task. However, balancing the optimization criteria of multi-task networks across different tasks is an area of active exploration. Here, we extend a previously proposed 3D attention-based network with four additional multi-task subnetworks for the detection of lung cancer and four auxiliary tasks (diagnosis of asthma, chronic bronchitis, chronic obstructive pulmonary disease, and emphysema). We introduce and evaluate a learning policy, Periodic Focusing Learning Policy (PFLP), that alternates the dominance of tasks throughout the training. To improve performance on the primary task, we propose an Internal-Transfer Weighting (ITW) strategy to suppress the loss functions on auxiliary tasks for the final stages of training. To evaluate this approach, we examined 3386 patients (single scan per patient) from the National Lung Screening Trial (NLST) and de-identified data from the Vanderbilt Lung Screening Program, with a 2517/277/592 (scans) split for training, validation, and testing. Baseline networks include a single-task strategy and a multi-task strategy without adaptive weights (PFLP/ITW), while primary experiments are multi-task trials with either PFLP or ITW or both. On the test set for lung cancer prediction, the baseline single-task network achieved prediction AUC of 0.8080 and multi-task baseline failed to converge (AUC 0.6720). However, applying PFLP helped multi-task network clarify and achieved test set lung cancer prediction AUC of 0.8402. Furthermore, our ITW technique boosted the PFLP enabled multi-task network and achieved an AUC of 0.8462 (McNemar test, p < 0.01). In conclusion, adaptive consideration of multi-task learning weights is important, and PFLP and ITW are promising strategies.
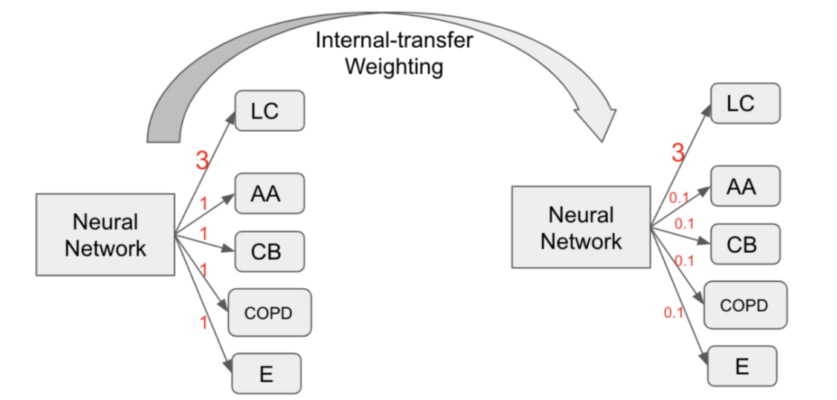