Abdominal Imaging
Motivation
Ventral hernia (VH) repair is one of the procedures most commonly performed by general surgeons worldwide, yet extensive variation exists in its delivery. Optimal candidates for repair, repair type, and ideal use of hernia mesh remain to be defined. There currently does not exist a widely accepted VH classification system to help identify disease severity and to improve outcomes. Even under optimal conditions, VHs occur in up to 28% of over 2 million patients undergoing abdominal operations each year. With current best-practices, VH repair is fraught with failure – recurrence rates ranging from 24-43% are reported. Recurrence of previously repaired VHs increases morbidity to patients and increases healthcare costs. Meanwhile, VHs continue to rise in incidence with nearly 350,000 repairs performed and total procedural costs for VH repair of $3.2 billion in the United States in 2006.
Most VH patients undergo computed tomography (CT) scanning to evaluate their abdominal wall. This information is used to make clinical judgments about a particular patient’s hernia for treatment and prognosis. Currently, these decisions are not evidence-based, but rather subjective and based mostly on expert opinion. We hypothesize that the CT datasets obtained from these studies are underutilized and provide a potentially rich — and automated — means of better characterizing VH.
Research objectives
- Develop and evaluate algorithms for the automated labeling of abdominal structures in patient populations using current generation clinically acquired CT data.
- Create new labeling paradigms so that automated methods can be efficiently learned from expertly labeled training data.
- Identify biomarkers based on structural imaging to improve accuracy of prognosis, guide treatment selection, and improve patient outcomes.
Progress
We were awarded a Vanderbilt Initiative in Surgery and Engineering (ViSE) pilot award to quantify VH and abdominal wall anatomy on clinical CT. We created the first methods to automatically identify the abdominal wall and characterize hernias. Through investigation of the abdominal wall, we realized the importance of having robust models of the underlying abdominal organs. We expanded collaborations to include Radiology collaborators to study liver and spleen pathologies. We applied our multi-atlas and machine learning expertise to create new methods to segment the abdominal organs and have shown leading accuracy for the spleen, an important biomarker for the response of liver cancer to drug therapy. We are translating these technologies for large-scale use in clinical trials. Meanwhile, we approached the problem from a theoretical standpoint and have created new constructs that better allow us to handle the large-scale sliding/shearing deformations present in abdominal anatomy.
Additionally, we integrated the organ segmentations into Vanderbilt Living In Virtual Environments (LIVE) lab lead and created a new course offering for spring 2013. We have IRB approval to conduct a study of surgeons and medical students using immersive virtual reality and are planning on applying these studies. We are integrating 3-D anatomy information to assist in patient communication, physician education, and surgical planning.
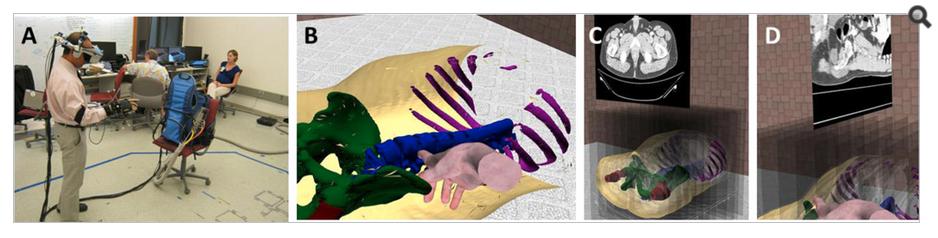