Foibles, Follies, and Fusion: Assessment of Statistical Label Fusion Techniques for Web-Based Collaborations using Minimal Training
Andrew J. Asman, Andrew G. Scoggins, Jerry L. Prince, Bennett A. Landman. “Foibles, Follies, and Fusion: Assessment of Statistical Label Fusion Techniques for Web-Based Collaborations using Minimal Training”, In Proceedings of the SPIE Medical Imaging Conference. Lake Buena Vista, Florida, February 2011 PMC3083117†
Full text: https://www.ncbi.nlm.nih.gov/pmc/articles/PMC3083117/
Abstract
Labeling or parcellation of structures of interest on magnetic resonance imaging (MRI) is essential in quantifying and characterizing correlation with numerous clinically relevant conditions. The use of statistical methods using automated methods or complete data sets from several different raters have been proposed to simultaneously estimate both rater reliability and true labels. An extension to these statistical based methodologies was proposed that allowed for missing labels, repeated labels and training trials. Herein, we present and demonstrate the viability of these statistical based methodologies using real world data contributed by minimally trained human raters. The consistency of the statistical estimates, the accuracy compared to the individual observations and the variability of both the estimates and the individual observations with respect to the number of labels are discussed. It is demonstrated that the Gaussian based statistical approach using the previously presented extensions successfully performs label fusion in a variety of contexts using data from online (Internet-based) collaborations among minimally trained raters. This first successful demonstration of a statistically based approach using “wild-type” data opens numerous possibilities for very large scale efforts in collaboration. Extension and generalization of these technologies for new application spaces will certainly present fascinating areas for continuing research.
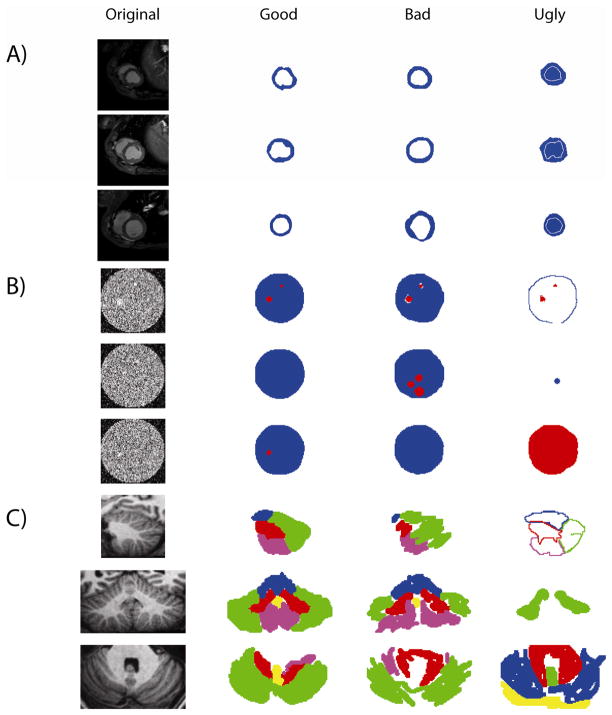