Statistical Fusion of Continuous Labels: Identification of Cardiac Landmarks
Fangxu Xing, Sahar Soleimanifard, Jerry L. Prince, Bennett A. Landman. “Statistical Fusion of Continuous Labels: Identification of Cardiac Landmarks”, In Proceedings of the SPIE Medical Imaging Conference. Lake Buena Vista, Florida, February 2011 (Oral Presentation) PMC3110005 †
Full text: https://www.ncbi.nlm.nih.gov/pmc/articles/PMC3110005/
Abstract
Image labeling is an essential task for evaluating and analyzing morphometric features in medical imaging data. Labels can be obtained by either human interaction or automated segmentation algorithms. However, both approaches for labeling suffer from inevitable error due to noise and artifact in the acquired data. The Simultaneous Truth And Performance Level Estimation (STAPLE) algorithm was developed to combine multiple rater decisions and simultaneously estimate unobserved true labels as well as each rater’s level of performance (i.e., reliability). A generalization of STAPLE for the case of continuous-valued labels has also been proposed. In this paper, we first show that with the proposed Gaussian distribution assumption, this continuous STAPLE formulation yields equivalent likelihoods for the bias parameter, meaning that the bias parameter—one of the key performance indices—is actually indeterminate. We resolve this ambiguity by augmenting the STAPLE expectation maximization formulation to include a priori probabilities on the performance level parameters, which enables simultaneous, meaningful estimation of both the rater bias and variance performance measures. We evaluate and demonstrate the efficacy of this approach in simulations and also through a human rater experiment involving the identification the intersection points of the right ventricle to the left ventricle in CINE cardiac data.
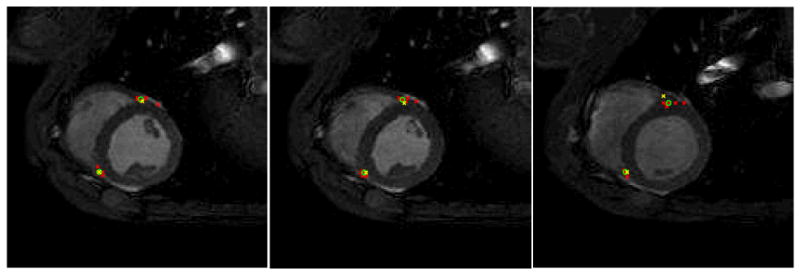