Collaborative Labeling of Malignant Glioma with WebMILL: A First Look
E. Singh, Z. Xu, A. Asman, L. Chambless, R. Thompson and B. Landman. “Collaborative Labeling of Malignant Glioma with WebMILL: A First Look.” In Proceedings of the SPIE Medical Imaging Conference. San Diego, California, February 2012 †
Abstract
Malignant gliomas are the most common form of primary neoplasm in the central nervous system, and one of the most rapidly fatal of all human malignancies. They are treated by maximal surgical resection followed by radiation and chemotherapy. Herein, we seek to improve the methods available to quantify the extent of tumors using newly presented, collaborative labeling techniques on magnetic resonance imaging. Traditionally, labeling medical images has entailed that expert raters operate on one image at a time, which is resource intensive and not practical for very large datasets. Using many, minimally trained raters to label images has the possibility of minimizing laboratory requirements and allowing high degrees of parallelism. A successful effort also has the possibility of reducing overall cost. This potentially transformative technology presents a new set of problems, because one must pose the labeling challenge in a manner accessible to people with little or no background in labeling medical images and raters cannot be expected to read detailed instructions. Hence, a different training method has to be employed. The training must appeal to all types of learners and have the same concepts presented in multiple ways to ensure that all the subjects understand the basics of labeling. Our overall objective is to demonstrate the feasibility of studying malignant glioma morphometry through statistical analysis of the collaborative efforts of many, minimally-trained raters. This study presents preliminary results on optimization of the WebMILL framework for neoplasm labeling and investigates the initial contributions of 78 raters labeling 98 whole-brain datasets.
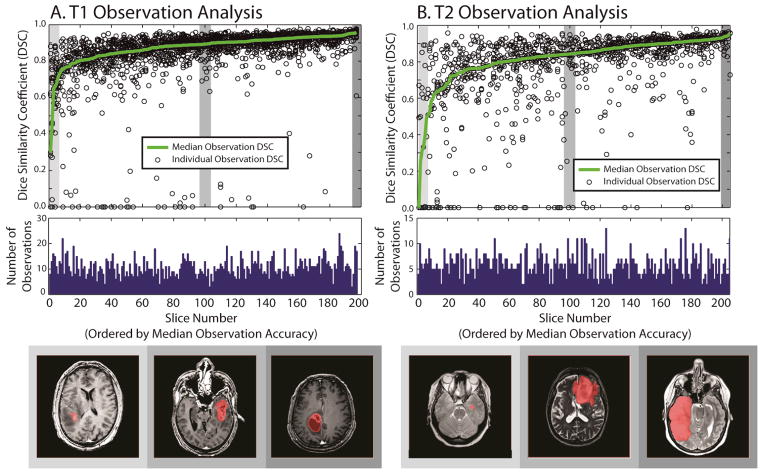
Illustration of minimally rater performance relative to an experienced rater for T1 data (A) and T2 data (B). The top row shows 200 slices which have been expertly labeled sorted by the median overlap with minimally trained raters. The center row shows the number of times each slice has been labeled. The final row illustrates an arbitrary example from the lowest, median, and highest accuracy slices.