Vanderbilt University Institute of Imaging Science Center for Computational Imaging XNAT: A multimodal data archive and processing environment
Robert L. Harrigan, Benjamin C. Yvernault, Brian D. Boyd, Stephen M. Damon, Kyla David Gibney, Benjamin N. Conrad, Nicholas S. Phillips, Baxter P. Rogers, Yurui Gao, Bennett A. Landman “Vanderbilt University Institute of Imaging Science Center for Computational Imaging XNAT: A multimodal data archive and processing environment” Neuroimage, 2014. In press May 2015†
Full Text: https://www.ncbi.nlm.nih.gov/pubmed/25988229
Abstract
The Vanderbilt University Institute for Imaging Science (VUIIS) Center for Computational Imaging (CCI) has developed a database built on XNAT housing over a quarter of a million scans. The database provides framework for (1) rapid prototyping, (2) large scale batch processing of images and (3) scalable project management. The system uses the web-based interfaces of XNAT and REDCap to allow for graphical interaction. A python middleware layer, the Distributed Automation for XNAT (DAX) package, distributes computation across the Vanderbilt Advanced Computing Center for Research and Education high performance computing center. All software are made available in open source for use in combining portable batch scripting (PBS) grids and XNAT servers.
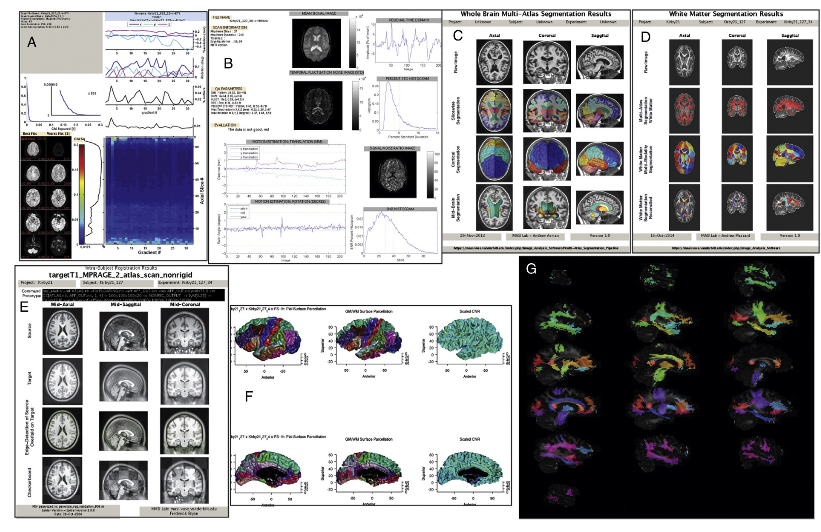