Histologically derived fiber response functions for diffusion MRI vary across white matter fibers-An ex vivo validation study in the squirrel monkey brain.
Kurt G. Schilling, Yurui Gao, Iwona Stepniewska, Vaibhav Janve, Bennett A. Landman, Adam W. Anderson. “Histologically derived fiber response functions for diffusion MRI vary across white matter fibers – an ex vivo validation study in the squirrel monkey brain”. NMR in Biomedicine. 2019 Jun;32(6):e4090. doi: 10.1002/nbm.4090. Epub 2019 Mar 25.
Full text: https://www.ncbi.nlm.nih.gov/pubmed/30908803
Abstract
Understanding the relationship between the diffusion-weighted MRI signal and the arrangement of white matter fibers is fundamental for accurate voxel-wise reconstruction of the fiber orientation distribution (FOD) and subsequent fiber tractography. Spherical deconvolution reconstruction techniques model the diffusion signal as the convolution of the FOD with a response function that represents the signal profile of a single fiber orientation. Thus, given the signal and a fiber response function, the FOD can be estimated in every imaging voxel by deconvolution. However, the selection of the appropriate response function remains relatively under-studied, and requires further validation. In this work, using 3D histologically defined FODs and the corresponding diffusion signal from three ex vivo squirrel monkey brains, we derive the ground truth response functions. We find that the histologically derived response functions differ from those conventionally used. Next, we find that response functions statistically vary across brain regions, which suggests that the practice of using the same kernel throughout the brain is not optimal. We show that different kernels lead to different FOD reconstructions, which in turn can lead to different tractography results depending on algorithmic parameters, with large variations in the accuracy of resulting reconstructions. Together, these results suggest there is room for improvement in estimating and understanding the relationship between the diffusion signal and the underlying FOD.
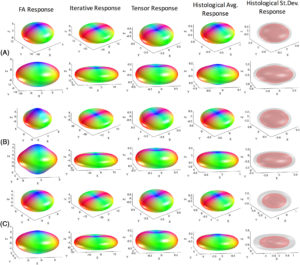