Deep learning reveals untapped information for local white-matter fiber reconstruction in diffusion-weighted MRI
Nath V, Schilling KG, Parvathaneni P, Hansen CB, Hainline AE, Huo Y, Blaber JA, Lyu I, Janve V, Gao Y, Stepniewska I, Anderson AW, Landman BA. Deep learning reveals untapped information for local white-matter fiber reconstruction in diffusion-weighted MRI. Magnetic resonance imaging. 2019 Oct 1;62:220-7.
Abstract
PURPOSE:
Diffusion-weighted magnetic resonance imaging (DW-MRI) is of critical importance for characterizing in-vivo white matter. Models relating microarchitecture to observed DW-MRI signals as a function of diffusion sensitization are the lens through which DW-MRI data are interpreted. Numerous modern approaches offer opportunities to assess more complex intra-voxel structures. Nevertheless, there remains a substantial gap between intra-voxel estimated structures and ground truth captured by 3-D histology.
METHODS:
Herein, we propose a novel data-driven approach to model the non-linear mapping between observed DW-MRI signals and ground truth structures using a sequential deep neural network regression using residual block deep neural network (ResDNN). Training was performed on two 3-D histology datasets of squirrel monkey brains and validated on a third. A second validation was performed using scan-rescan datasets of 12 subjects from Human Connectome Project. The ResDNN was compared with multiple micro-structure reconstruction methods and super resolved-constrained spherical deconvolution (sCSD) in particular as baseline for both the validations.
RESULTS:
Angular correlation coefficient (ACC) is a correlation/similarity measure and can be interpreted as accuracy when compared with a ground truth. The median ACC of ResDNN is 0.82 and median ACC’s of different variants of CSD are 0.75, 0.77, 0.79. The mean, median and std. of ResDNN & sCSD ACC across 12 subjects from HCP are 0.74, 0.88, 0.31 and 0.61, 0.71, 0.31 respectively.
CONCLUSION:
This work highlights the ability of deep learning to capture linkages between ex-vivo ground truth data with feasible MRI sequences. The data-driven approach is applicable to human in-vivo data and results in intriguingly high reproducibility of orientation structure.
Full text: https://www.ncbi.nlm.nih.gov/pubmed/31323317
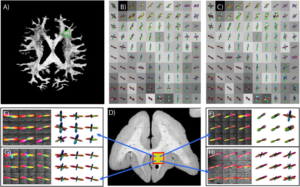