Empirical field mapping for gradient nonlinearity correction of multi-site diffusion weighted MRI
Colin B. Hansen, Baxter P. Rogers, Kurt G. Schilling, Vishwesh Nath, Justin A. Blaber, Okan Irfanoglu, Alan Barnett, Carlo Pierpaoli, Adam W. Anderson, Bennett A. Landman. “Empirical field mapping for gradient nonlinearity correction of multi-site diffusion weighted MRI.” Magnetic Resonance Imaging 2020.
Abstract
Background: Achieving inter-site / inter-scanner reproducibility of diffusion weighted magnetic resonance imaging (DW-MRI) metrics has been challenging given differences in acquisition protocols, analysis models, and hardware factors.
Purpose: Magnetic field gradients impart scanner-dependent spatial variations in the applied diffusion weighting that can be corrected if the gradient nonlinearities are known. However, retrieving manufacturer nonlinearity specifications is not well supported and may introduce errors in interpretation of units or coordinate systems. We propose an empirical approach to mapping the gradient nonlinearities with sequences that are supported across the major scanner vendors.
Study Type: Prospective observational study
Subjects: A spherical isotropic diffusion phantom, and a single human control volunteer
Field Strength/Sequence: 3T (two scanners). Stejskal-Tanner spin echo sequence with b-values of 1000, 2000 s/mm2 with 12, 32, and 384 diffusion gradient directions per shell.
Assessment: We compare the proposed correction with the prior approach using manufacturer specifications against typical diffusion pre-processing pipelines (i.e., ignoring spatial gradient nonlinearities). In phantom data, we evaluate metrics against the ground truth. In human and phantom data, we evaluate reproducibility across scans, sessions, and hardware.
Statistical Tests: Wilcoxon rank-sum test between uncorrected and corrected data.
Results: In phantom data, our correction method reduces variation in mean diffusivity across sessions over uncorrected data (p<0.05). In human data, we show that this method can also reduce variation in mean diffusivity across scanners (p<0.05).
Conclusion: Our method is relatively simple, fast, and can be applied retroactively. We advocate incorporating voxel-specific b-value and b-vector maps should be incorporated in DW-MRI harmonization preprocessing pipelines to improve quantitative accuracy of measured diffusion parameters.
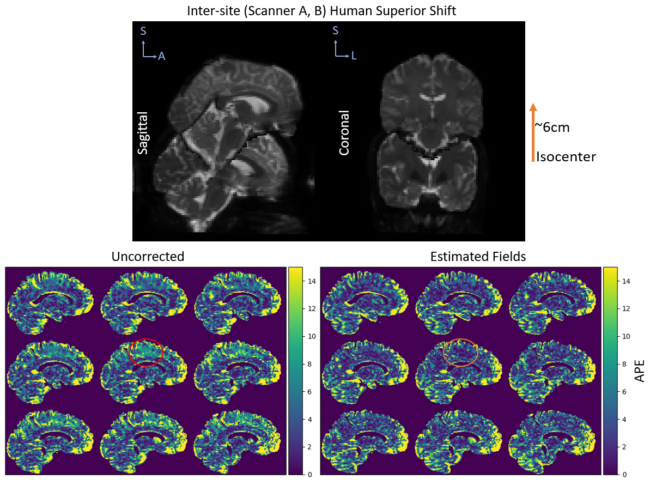