Texture Analysis Improves Level Set Segmentation of the Anterior Abdominal Wall.
Zhoubing Xu, Wade M. Allen, Rebeccah B. Baucom, Benjamin K. Poulose, Bennett A. Landman. “Texture Analysis Improves Level Set Segmentation of the Anterior Abdominal Wall.” Medical Physics. 2013 Dec;40(12):121901. † PMC3838426
Full Text: https://www.ncbi.nlm.nih.gov/pubmed/24320512
Abstract
PURPOSE:
The treatment of ventral hernias (VH) has been a challenging problem for medical care. Repair of these hernias is fraught with failure; recurrence rates ranging from 24% to 43% have been reported, even with the use of biocompatible mesh. Currently, computed tomography (CT) is used to guide intervention through expert, but qualitative, clinical judgments, notably, quantitative metrics based on image-processing are not used. The authors propose that image segmentation methods to capture the three-dimensional structure of the abdominal wall and its abnormalities will provide a foundation on which to measure geometric properties of hernias and surrounding tissues and, therefore, to optimize intervention.
METHODS:
In this study with 20 clinically acquired CT scans on postoperative patients, the authors demonstrated a novel approach to geometric classification of the abdominal. The authors’ approach uses a texture analysis based on Gabor filters to extract feature vectors and follows a fuzzy c-means clustering method to estimate voxelwise probability memberships for eight clusters. The memberships estimated from the texture analysis are helpful to identify anatomical structures with inhomogeneous intensities. The membership was used to guide the level set evolution, as well as to derive an initial start close to the abdominal wall.
RESULTS:
Segmentation results on abdominal walls were both quantitatively and qualitatively validated with surface errors based on manually labeled ground truth. Using texture, mean surface errors for the outer surface of the abdominal wall were less than 2 mm, with 91% of the outer surface less than 5 mm away from the manual tracings; errors were significantly greater (2-5 mm) for methods that did not use the texture.
CONCLUSIONS:
The authors’ approach establishes a baseline for characterizing the abdominal wall for improving VH care. Inherent texture patterns in CT scans are helpful to the tissue classification, and texture analysis can improve the level set segmentation around the abdominal region.
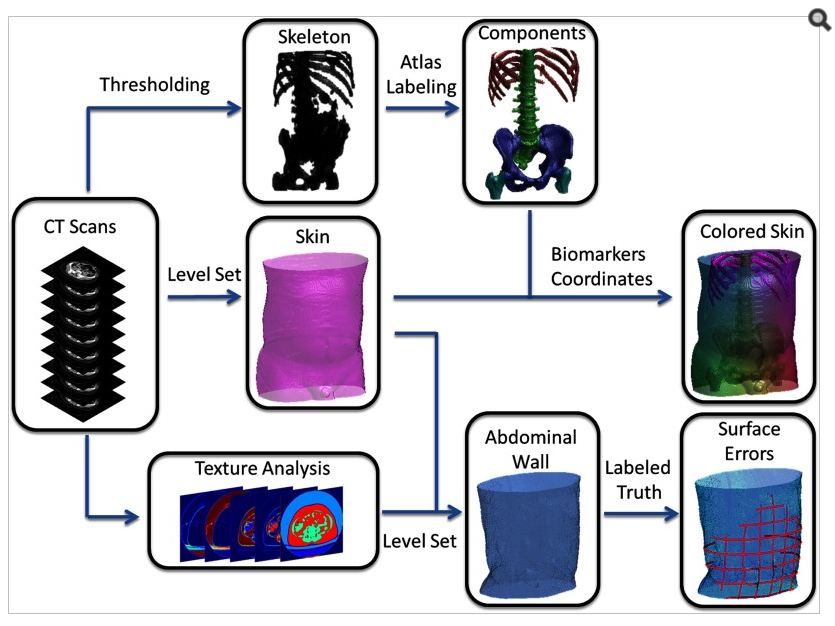