Foibles, Follies, and Fusion: Web-Based Collaboration for Medical Image Labeling
Bennett A Landman, Andrew J Asman, Andrew G Scoggins, John A Bogovic, Joshua A Stein; Jerry L Prince, “Foibles, Follies, and Fusion: Web-Based Collaboration for Medical Image Labeling”, NeuroImage. 2012 Jan 2;59(1):530-9. PMC3195954 †
Full text: https://www.ncbi.nlm.nih.gov/pmc/articles/PMC3195954/
Abstract
Labels that identify specific anatomical and functional structures within medical images are essential to the characterization of the relationship between structure and function in many scientific and clinical studies. Automated methods that allow for high throughput have not yet been developed for all anatomical targets or validated for exceptional anatomies, and manual labeling remains the gold standard in many cases. However, manual placement of labels within a large image volume such as that obtained using magnetic resonance imaging is exceptionally challenging, resource intensive, and fraught with intra- and inter-rater variability. The use of statistical methods to combine labels produced by multiple raters has grown significantly in popularity, in part, because it is thought that by estimating and accounting for rater reliability estimates of the true labels will be more accurate. This paper demonstrates the performance of a class of these statistical label combination methodologies using real-world data contributed by minimally trained human raters. The consistency of the statistical estimates, the accuracy compared to the individual observations, and the variability of both the estimates and the individual observations with respect to the number of labels are presented. It is demonstrated that statistical fusion successfully combines label information using data from online (Internet-based) collaborations among minimally trained raters. This first successful demonstration of a statistically based approach using minimally trained raters opens numerous possibilities for very large scale efforts in collaboration. Extension and generalization of these technologies for new applications will certainly present fascinating areas for continuing research.
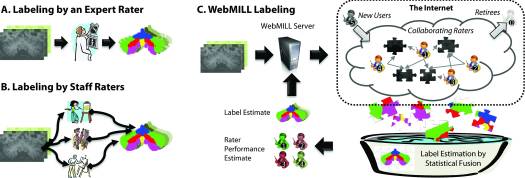