Formulating Spatially Varying Performance in the Statistical Fusion Framework
Andrew J. Asman and Bennett A. Landman, “Formulating Spatially Varying Performance in the Statistical Fusion Framework”, IEEE Transactions on Medical Imaging. 2012 Jun;31(6):1326-36. PMC3368083 †
Full text: https://www.ncbi.nlm.nih.gov/pmc/articles/PMC3368083/
Abstract
To date, label fusion methods have primarily relied either on global (e.g. STAPLE, globally weighted vote) or voxelwise (e.g. locally weighted vote) performance models. Optimality of the statistical fusion framework hinges upon the validity of the stochastic model of how a rater errs (i.e., the labeling process model). Hitherto, approaches have tended to focus on the extremes of potential models. Herein, we propose an extension to the STAPLE approach to seamlessly account for spatially varying performance by extending the performance level parameters to account for a smooth, voxelwise performance level field that is unique to each rater. This approach, Spatial STAPLE, provides significant improvements over state-of-the-art label fusion algorithms in both simulated and empirical </h2>data sets.
Keywords: STAPLE, Spatial STAPLE, Rater Models, Statistical Fusion, Multi-Atlas Segmentation
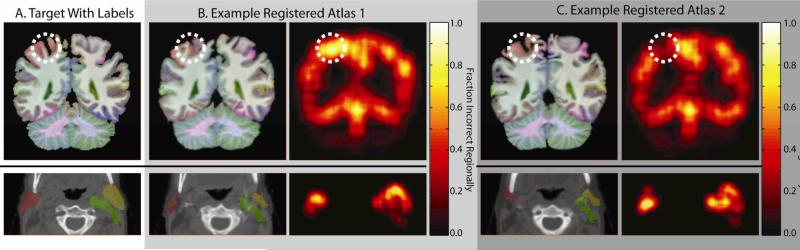