Self-Supervised Pre-Training of Swin Transformers for 3D Medical Image Analysis
Abstract
Vision Transformers (ViT)s have shown great performance in self-supervised learning of global and local representations that can be transferred to downstream applications. Inspired by these results, we introduce a novel self-supervised learning framework with tailored proxy tasks for medical image analysis. Specifically, we propose: (i) a new 3D transformer-based model, dubbed Swin UNEt TRansformers (Swin UNETR), with a hierarchical encoder for self-supervised pre-training; (ii) tailored proxy tasks for learning the underlying pattern of human anatomy. We demonstrate successful pre-training of the proposed model on 5,050 publicly available computed tomography (CT) images from various body organs. The effectiveness of our approach is validated by fine-tuning the pre-trained models on the Beyond the Cranial Vault (BTCV) Segmentation Challenge with 13 abdominal organs and segmentation tasks from the Medical Segmentation Decathlon (MSD) dataset. Our model is currently the state-of-the-art on the public test leaderboards of both MSD and BTCV datasets. Code: https://monai.io/research/swin unetr.
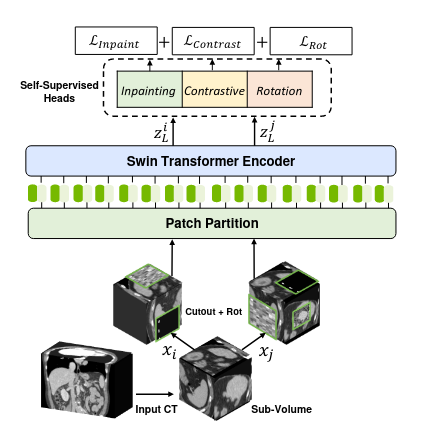
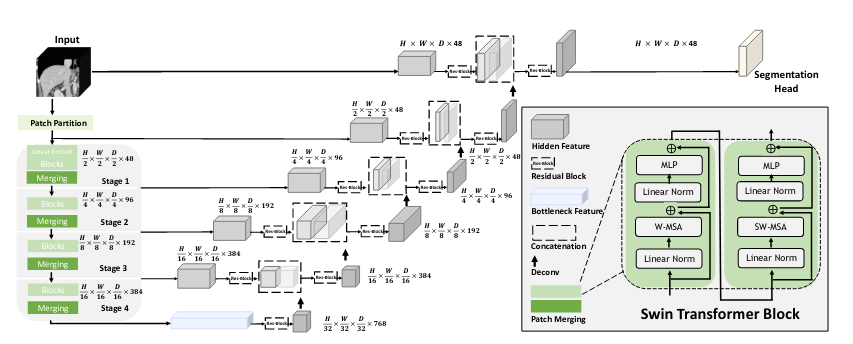