Out-of-Atlas Labeling: A Multi-Atlas Approach to Cancer Segmentation
Andrew J. Asman and Bennett A. Landman, “Out-of-Atlas Labeling: A Multi-Atlas Approach to Cancer Segmentation”, In Proceedings of the 2012 International Symposium on Biomedical Imaging (ISBI). Barcelona, Spain†
Full Text: https://www.ncbi.nlm.nih.gov/pmc/articles/PMC3892947/
Abstract
Conventional automated segmentation techniques for magnetic resonance imaging (MRI) fail to perform in a robust and consistent manner when brain anatomy differs wildly from expectations – as is often the case in brain cancers. We propose a novel out-of-atlas technique to estimate the spatial extent of abnormal brain regions by combining multi-atlas based segmentation with semi-local non-parametric intensity analysis. In a study with 30 clinically-acquired MRI scans of patients with malignant gliomas and 29 atlases of normal anatomy from research acquisitions, we demonstrate that this technique robustly identifies cancerous regions. The resulting segmentations could be used to study cancer morphometrics or guide selection/application/refinement of tumor analysis models or regional image quantification approaches.
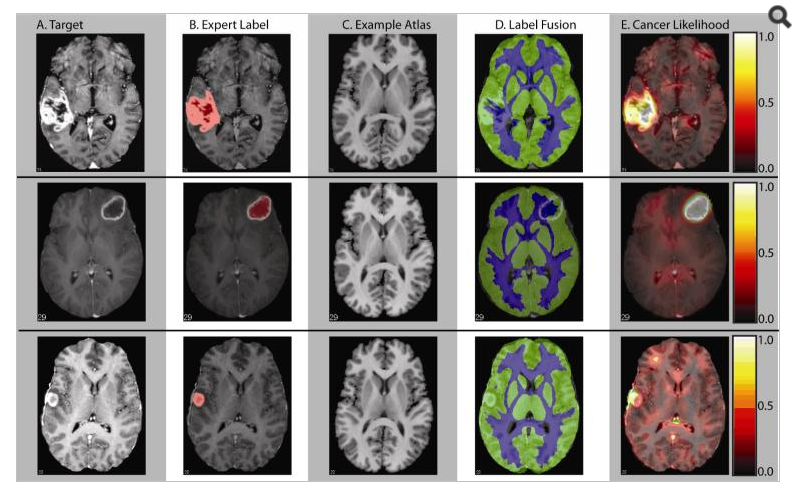