Robust GM/WM Segmentation of the Spinal Cord with Iterative Non-Local Statistical Fusion
Andrew J. Asman, Seth A. Smith, Daniel Reich, and Bennett A. Landman. “Robust GM/WM Segmentation of the Spinal Cord with Iterative Non-Local Statistical Fusion”, In Proceedings of the International Conference on Medical Image Computing and Computer Assisted Intervention (MICCAI). 2013 Nagoya, Japan
Full text: https://www.ncbi.nlm.nih.gov/pmc/articles/PMC3918679/
Abstract
New magnetic resonance imaging (MRI) sequences are enabling clinical study of the in vivo spinal cord’s internal structure. Yet, low contrast-to-noise ratio, artifacts, and imaging distortions have limited the applicability of tissue segmentation techniques pioneered elsewhere in the central nervous system. Recently, methods have been presented for cord/non-cord segmentation on MRI and the feasibility of gray matter/white matter tissue segmentation has been evaluated. To date, no automated algorithms have been presented. Herein, we present a non-local multi-atlas framework that robustly identifies the spinal cord and segments its internal structure with submillimetric accuracy. The proposed algorithm couples non-local fusion with a large number of slice-based atlases (as opposed to typical volumetric ones). To improve performance, the fusion process is interwoven with registration so that segmentation information guides registration and vice versa. We demonstrate statistically significant improvement over state-of-the-art benchmarks in a study of 67 patients. The primary contributions of this work are (1) innovation in non-volumetric atlas information, (2) advancement of label fusion theory to include iterative registration/segmentation, and (3) the first fully automated segmentation algorithm for spinal cord internal structure on MRI.
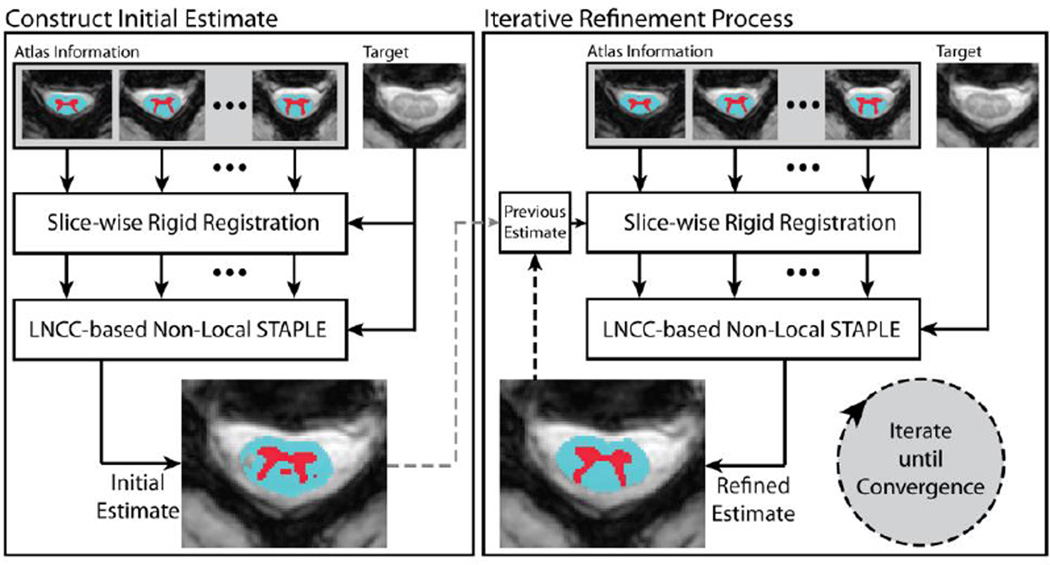