Robust Estimation of Spatially Variable Noise Fields
B. A. Landman, P-L Bazin, S. A. Smith, and J. L. Prince, “Robust Estimation of Spatially Variable Noise Fields”, Magnetic Resonance in Medicine, Aug;62(2):500-9. 2009 PMC2806192
Full text: https://www.ncbi.nlm.nih.gov/pmc/articles/PMC2806192/
Abstract
Consideration of spatially variable noise fields is becoming increasing necessary in magnetic resonance imaging given recent innovations in artifact identification and statistically-driven image processing. Fast imaging methods enable study of difficult anatomical targets and improve image quality but also increase the spatial variability in the noise field. Traditional analysis techniques have either assumed that the noise is constant across the field of view (or region of interest) or have relied on separate magnetic resonance image acquisitions to measure the noise field. These methods are either inappropriate for many modern scanning protocols or are overly time-consuming for already lengthy scanning sessions. We propose a new, general framework for estimating spatially variable noise fields from related, but independent magnetic resonance scans which we call noise field equivalent scans. These heuristic analyses enable robust noise field estimation in the presence of artifacts. Generalization of noise estimators based on uniform regions, difference images, and maximum likelihood are presented and compared with the estimators derived from the proposed framework. Simulations of diffusion tensor imaging and T2-relaxometry demonstrate a ten-fold reduction in mean squared error in noise field estimation, and these improvements are shown to be robust to artifact contamination. In vivo studies show that spatially variable noise fields can be readily estimated with typical data acquired at 1.5T.
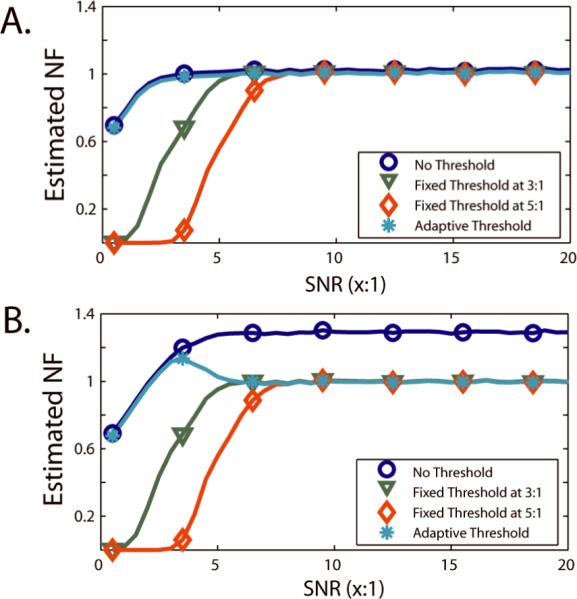