Biological Parametric Mapping WITH Robust AND Non-Parametric Statistics
Xue Yang, Lori Beason-Held, Susan M Resnick, Bennett A Landman. “Biological Parametric Mapping with Robust and Non-Parametric Statistics”, NeuroImage 57 (2011) Jul 423–430 PMC3114289†
Full text: https://www.ncbi.nlm.nih.gov/pmc/articles/PMC3114289/
Abstract
Mapping the quantitative relationship between structure and function in the human brain is an important and challenging problem. Numerous volumetric, surface, regions of interest and voxelwise image processing techniques have been developed to statistically assess potential correlations between imaging and non-imaging metrices. Recently, biological parametric mapping has extended the widely popular statistical parametric mapping approach to enable application of the general linear model to multiple image modalities (both for regressors and regressands) along with scalar valued observations. This approach offers great promise for direct, voxelwise assessment of structural and functional relationships with multiple imaging modalities. However, as presented, the biological parametric mapping approach is not robust to outliers and may lead to invalid inferences (e.g., artifactual low p-values) due to slight mis-registration or variation in anatomy between subjects. To enable widespread application of this approach, we introduce robust regression and non-parametric regression in the neuroimaging context of application of the general linear model. Through simulation and empirical studies, we demonstrate that our robust approach reduces sensitivity to outliers without substantial degradation in power. The robust approach and associated software package provide a reliable way to quantitatively assess voxelwise correlations between structural and functional neuroimaging modalities.
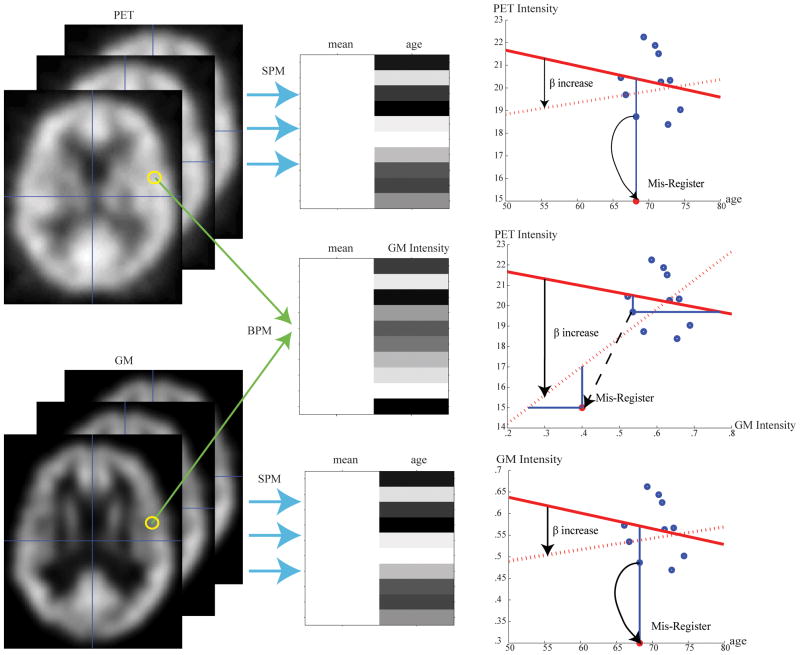