Simultaneous Segmentation and Statistical Label Fusion.
A. Asman and B. Landman. “Simultaneous Segmentation and Statistical Label Fusion.” In Proceedings of the SPIE Medical Imaging Conference. San Diego, California, February 2012 (Oral Presentation) †
Full Text: https://www.ncbi.nlm.nih.gov/pubmed/24357909
Abstract
Labeling or segmentation of structures of interest in medical imaging plays an essential role in both clinical and scientific understanding. Two of the common techniques to obtain these labels are through either fully automated segmentation or through multi-atlas based segmentation and label fusion. Fully automated techniques often result in highly accurate segmentations but lack the robustness to be viable in many cases. On the other hand, label fusion techniques are often extremely robust, but lack the accuracy of automated algorithms for specific classes of problems. Herein, we propose to perform simultaneous automated segmentation and statistical label fusion through the reformulation of a generative model to include a linkage structure that explicitly estimates the complex global relationships between labels and intensities. These relationships are inferred from the atlas labels and intensities and applied to the target using a non-parametric approach. The novelty of this approach lies in the combination of previously exclusive techniques and attempts to combine the accuracy benefits of automated segmentation with the robustness of a multi-atlas based approach. The accuracy benefits of this simultaneous approach are assessed using a multi-label multi- atlas whole-brain segmentation experiment and the segmentation of the highly variable thyroid on computed tomography images. The results demonstrate that this technique has major benefits for certain types of problems and has the potential to provide a paradigm shift in which the lines between statistical label fusion and automated segmentation are dramatically blurred.
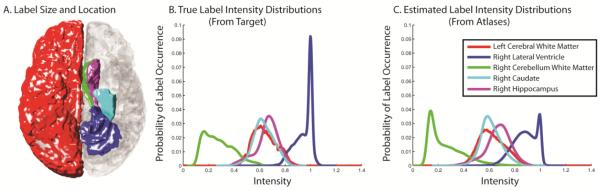