Robust Inter-Modality Multi-Atlas Segmentation for PACS-based DTI Quality Control
Andrew J. Asman, Carolyn B. Lauzon, Bennett A. Landman. “Robust Inter-Modality Multi-Atlas Segmentation for PACS-based DTI Quality Control.” In Proceedings of the SPIE Medical Imaging Conference. Orlando, Florida, February 2013. Oral Presentation. †
Full text: https://www.ncbi.nlm.nih.gov/pmc/articles/PMC3874255/
Abstract
Anatomical contexts (spatial labels) are critical for interpretation of medical imaging content. Numerous approaches have been devised for segmentation, query, and retrieval within the Picture Archive and Communication System (PACS) framework. To date, application-based methods for anatomical localization and tissue classification have yielded the most successful results, but these approaches typically rely upon the availability of standardized imaging sequences. With the ever expanding scope of PACS archives — including multiple imaging modalities, multiple image types within a modality, and multi-site efforts, it is becoming increasingly burdensome to devise a specific method for each data type. To address the challenge of generalizing segmentations from one modality to another, we consider multi-atlas segmentation to transfer label information from labeled T1-weighted MRI data to unlabeled B0 data collected in a diffusion tensor imaging (DTI) experiment. The label transfer approach is fully automated and enables a generalizable cross-modality segmentation method. Herein, we propose a multi-tier multi-atlas segmentation framework for the segmentation of previously unlabeled imaging modalities (e.g., B0images for DTI analysis). We show that this approach can be used to construct informed structure-wise noise estimates for fractional anisotropy (FA) measurements of DTI. Although this label transfer methodology is demonstrated in the context of quality control of DTI images, the proposed framework is applicable to any application where the segmentation of unlabeled modalities is limited due to the current collection of available atlases.
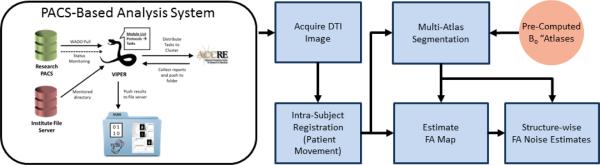