Robust Non-Local Multi-Atlas Segmentation of the Optic Nerve
A. Asman, Michael P. DeLisi, Louise A. Mawn, Robert L. Galloway, and B. Landman. “Robust Non-Local Multi-Atlas Segmentation of the Optic Nerve.” In Proceedings of the SPIE Medical Imaging Conference. Orlando, Florida, February 2013. Oral Presentation. † PMCID 24478826
Full text: https://www.ncbi.nlm.nih.gov/pmc/articles/PMC3903299/?report=classic
Abstract
Labeling or segmentation of structures of interest on medical images plays an essential role in both clinical and scientific understanding of the biological etiology, progression, and recurrence of pathological disorders. Here, we focus on the optic nerve, a structure that plays a critical role in many devastating pathological conditions – including glaucoma, ischemic neuropathy, optic neuritis and multiple-sclerosis. Ideally, existing fully automated procedures would result in accurate and robust segmentation of the optic nerve anatomy. However, current segmentation procedures often require manual intervention due to anatomical and imaging variability. Herein, we propose a framework for robust and fully-automated segmentation of the optic nerve anatomy. First, we provide a robust registration procedure that results in consistent registrations, despite highly varying data in terms of voxel resolution and image field-of-view. Additionally, we demonstrate the efficacy of a recently proposed non-local label fusion algorithm that accounts for small scale errors in registration correspondence. On a dataset consisting of 31 highly varying computed tomography (CT) images of the human brain, we demonstrate that the proposed framework consistently results in accurate segmentations. In particular, we show (1) that the proposed registration procedure results in robust registrations of the optic nerve anatomy, and (2) that the non-local statistical fusion algorithm significantly outperforms several of the state-of-the-art label fusion algorithms.
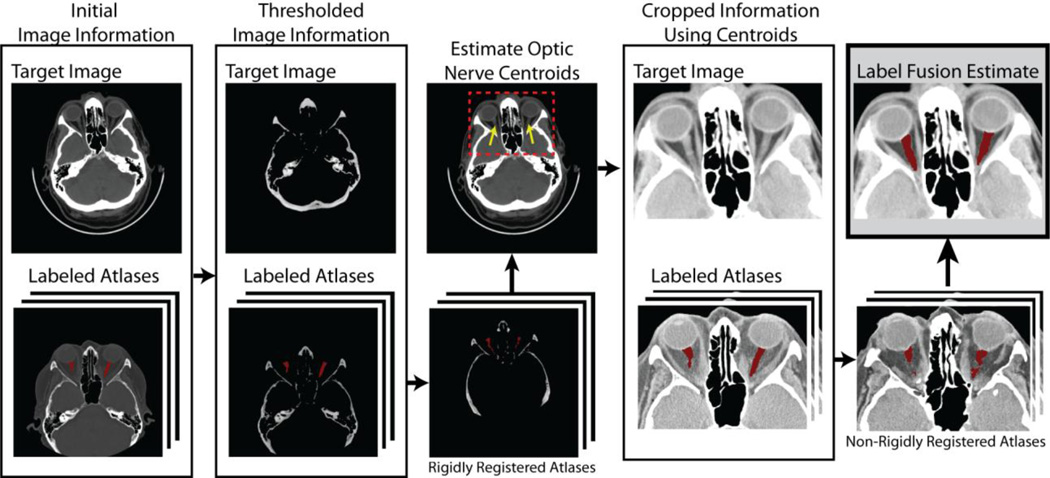