Robust Optic Nerve Segmentation on Clinically Acquired CT
Swetasudha Panda, Andrew J. Asman, Michael P. DeLis, Louise A. Mawn, Robert L. Galloway, Bennett A. Landman. “Robust Optic Nerve Segmentation on Clinically Acquired CT.” In Proceedings of the SPIE Medical Imaging Conference. San Diego, California, February 2014. Oral Presentation. †
Full Text: https://www.ncbi.nlm.nih.gov/pubmed/24817810
Abstract
The optic nerve is a sensitive central nervous system structure, which plays a critical role in many devastating pathological conditions. Several methods have been proposed in recent years to segment the optic nerve automatically, but progress toward full automation has been limited. Multi-atlas methods have been successful for brain segmentation, but their application to smaller anatomies remains relatively unexplored. Herein we evaluate a framework for robust and fully automated segmentation of the optic nerves, eye globes and muscles. We employ a robust registration procedure for accurate registrations, variable voxel resolution and image field-of-view. We demonstrate the efficacy of an optimal combination of SyN registration and a recently proposed label fusion algorithm (Non-local Spatial STAPLE) that accounts for small-scale errors in registration correspondence. On a dataset containing 30 highly varying computed tomography (CT) images of the human brain, the optimal registration and label fusion pipeline resulted in a median Dice similarity coefficient of 0.77, symmetric mean surface distance error of 0.55 mm, symmetric Hausdorff distance error of 3.33 mm for the optic nerves. Simultaneously, we demonstrate the robustness of the optimal algorithm by segmenting the optic nerve structure in 316 CT scans obtained from 182 subjects from a thyroid eye disease (TED) patient population.
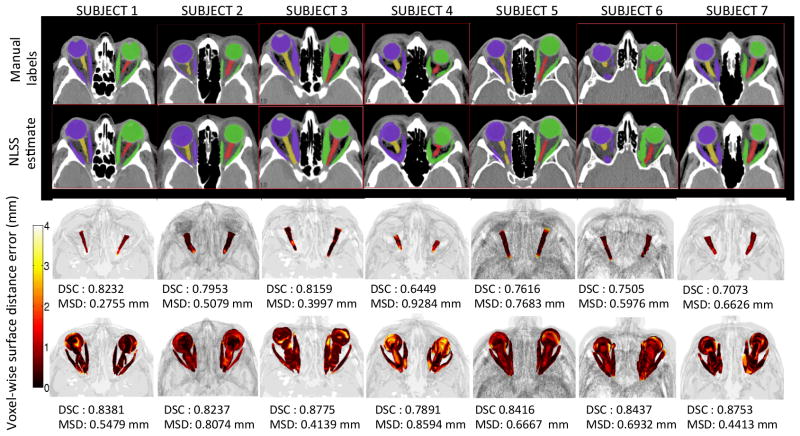
Qualitative results for the optimal multi-atlas segmentation approach for 7 subjects are shown. For a typical subject, the top rows compare manual and automatic results for a representative 2D slice. The bottom rows show point-wise surface distance error of the label fusion estimate for the ONs and the eye globe structure. The proposed multi-atlas pipeline results in reasonably accurate segmentations for the ON structure. However, slight over segmentations of the ONs can be observed in certain cases (subjects 4 and 7).