Structural Functional Associations of the Orbit in Thyroid Eye Disease: Kalman Filters to Track Extraocular Muscles
Shikha Chaganti, Katrina Nelson, Kevin Mundy, Yifu Luo, Robert L. Harrigan, Steve Damon, Daniel Fabbri, Louise Mawn, Bennett Landman. “Structural Functional Associations of the Orbit in Thyroid Eye Disease: Kalman Filters to Track Extraocular Muscles”. In Proceedings of the SPIE Medical Imaging Conference. San Diego, California, February 2016. Oral presentation.
Full text: https://www.ncbi.nlm.nih.gov/pmc/articles/PMC4845964/
Abstract
Pathologies of the optic nerve and orbit impact millions of Americans and quantitative assessment of the orbital structures on 3-D imaging would provide objective markers to enhance diagnostic accuracy, improve timely intervention and eventually preserve visual function. Recent studies have shown that the multi-atlas methodology is suitable for identifying orbital structures, but challenges arise in the identification of the individual extraocular rectus muscles that control eye movement. This is increasingly problematic in diseased eyes, where these muscles often appear to fuse at the back of the orbit (at the resolution of clinical computed tomography imaging) due to inflammation or crowding. We propose the use of Kalman filters to track the muscles in three-dimensions to refine multi-atlas segmentation and resolve ambiguity due to imaging resolution, noise, and artifacts. The purpose of our study is to investigate a method of automatically generating orbital metrics from CT imaging and demonstrate the utility of the approach by correlating structural metrics of the eye orbit with clinical data and visual function measures in subjects with thyroid eye disease. The pilot study demonstrates that automatically calculated orbital metrics are strongly correlated with several clinical characteristics. Moreover, the superior, inferior, medial and lateral rectus muscles obtained using Kalman filters are each correlated with different categories of functional deficit. These findings serve as foundation for further investigation in the use of CT imaging in the study, analysis and diagnosis of ocular diseases, specifically thyroid eye disease.
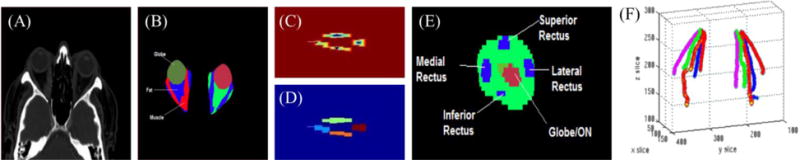