Towards Machine Learning Prediction of Deep Brain Stimulation (DBS) Intra-operative Efficacy Maps
Bermudez, C., Rodriguez, W., Huo, Y., Hainline, A. E., Li, R., Shults, R., … & Landman, B. A. (2018). Towards Machine Learning Prediction of Deep Brain Stimulation (DBS) Intra-operative Efficacy Maps. arXiv preprint arXiv:1811.10415.
Full Text: https://arxiv.org/abs/1811.10415
Abstract
Deep brain stimulation (DBS) has the potential to improve the quality of life of people with a variety of neurological diseases. A key challenge in DBS is in the placement of a stimulation electrode in the anatomical location that maximizes efficacy and minimizes side effects. Pre-operative localization of the optimal stimulation zone can reduce surgical times and morbidity. Current methods of producing efficacy probability maps follow an anatomical guidance on magnetic resonance imaging (MRI) to identify the areas with the highest efficacy in a population. In this work, we propose to revisit this problem as a classification problem, where each voxel in the MRI is a sample informed by the surrounding anatomy. We use a patch-based convolutional neural network to classify a stimulation coordinate as having a positive reduction in symptoms during surgery. We use a cohort of 187 patients with a total of 2,869 stimulation coordinates, upon which 3D patches were extracted and associated with an efficacy score. We compare our results with a registration-based method of surgical planning. We show an improvement in the classification of intraoperative stimulation coordinates as a positive response in reduction of symptoms with AUC of 0.670 compared to a baseline registration-based approach, which achieves an AUC of 0.627 (p < 0.01). Although additional validation is needed, the proposed classification framework and deep learning method appear well-suited for improving pre-surgical planning and personalize treatment strategies.
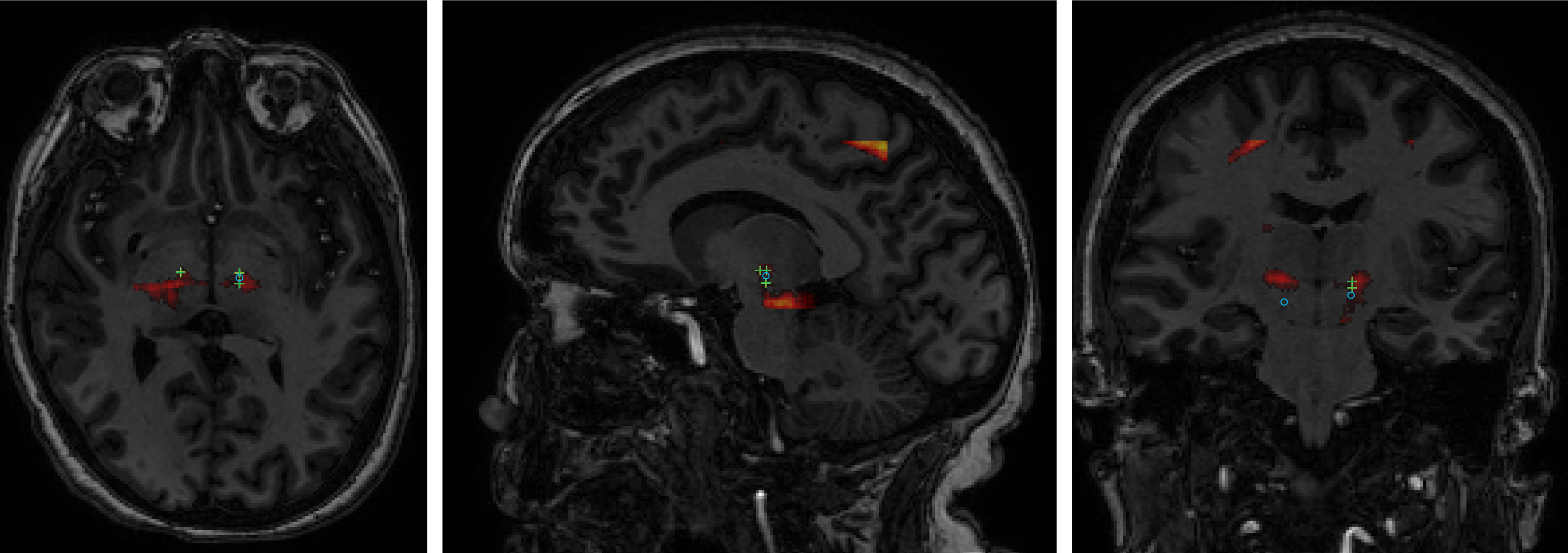