Improved gray matter surface based spatial statistics in neuroimaging studies
Neuroimaging often involves acquiring high-resolution anatomical images along with other low-resolution image modalities, like diffusion and functional magnetic resonance imaging. Performing gray matter statistics with low-resolution image modalities is a challenge due to registration artifacts and partial volume effects. Gray matter surface based spatial statistics (GS-BSS) has been shown to provide higher sensitivity using gray matter surfaces compared to that of skeletonization approach of gray matter based spatial statistics which is adapted from tract based spatial statistics in diffusion studies. In this study, we improve upon GS-BSS incorporating neurite orientation dispersion and density imaging (NODDI) based search (denoted N-GSBSS) by 1) enhancing metrics mapping from native space, 2) incorporating maximum orientation dispersion index (ODI) search along surface normal, and 3) proposing applicability to other modalities, such as functional MRI (fMRI). We evaluated the performance of N-GSBSS against three baseline pipelines: volume-based registration, FreeSurfer’s surface registration and ciftify pipeline for fMRI and simulation studies. First, qualitative mean ODI results are shown for N-GSBSS with and without NODDI based search in comparision with ciftify pipeline. Second, we conducted one-sample t-tests on working memory activations in fMRI to show that the proposed method can aid in the analysis of low resolution fMRI data. Finally we performed a sensitivity test in a simulation study by varying percentage change of intensity values within a region of interest in gray matter probability maps. N-GSBSS showed higher sensitivity in the simulation test compared to the other methods capturing difference between the groups starting at 10 percent change in the intensity values. The computational time of N-GSBSS is 68 times faster than that of traditional surface-based or 86 times faster than that of ciftify pipeline analysis.
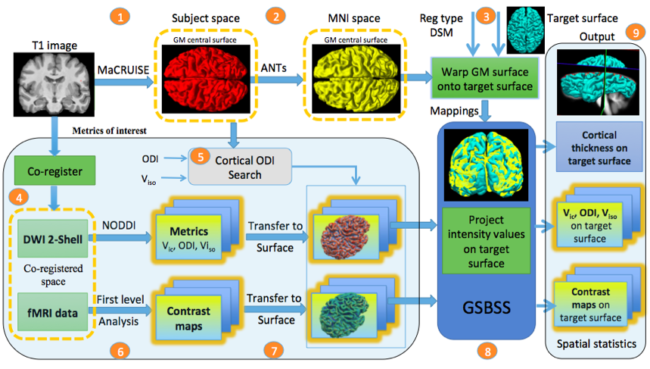
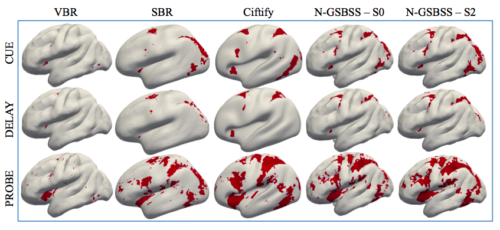