Learning from dispersed manual annotations with an optimized data weighting policy
Yucheng Tang, Riqiang Gao, Yunqiang Chen, Dashan Gao, Michael R. Savona, Richard G. Abramson, Shunxing Bao, Yuankai Huo and Bennett A. Landman, “Learning from Dispersed Manual Annotations with an Optimized Data Weighting Policy”, Journal of Medical Imaging, 2020.
Full Text:
Abstract
https://pubmed.ncbi.nlm.nih.gov/32775501/
Purpose: Deep learning methods have become essential tools for quantitative interpretation of
medical imaging data, but training these approaches is highly sensitive to biases and class imbalance
in the available data. There is an opportunity to increase the available training data by
combining across different data sources (e.g., distinct public projects); however, data collected
under different scopes tend to have differences in class balance, label availability, and subject
demographics. Recent work has shown that importance sampling can be used to guide training
selection. To date, these approaches have not considered imbalanced data sources with distinct
labeling protocols.
Approach: We propose a sampling policy, known as adaptive stochastic policy (ASP), inspired
by reinforcement learning to adapt training based on subject, data source, and dynamic use
criteria. We apply ASP in the context of multiorgan abdominal computed tomography segmentation.
Training was performed with cross validation on 840 subjects from 10 data sources.
External validation was performed with 20 subjects from 1 data source.
Results: Four alternative strategies were evaluated with the state-of-the-art baseline as upper
confident bound (UCB). ASP achieves average Dice of 0.8261 compared to 0.8135 UCB
(p < 0.01, paired t-test) across fivefold cross validation. On withheld testing datasets, the proposed
ASP achieved 0.8265 mean Dice versus 0.8077 UCB (p < 0.01, paired t-test).
Conclusions: ASP provides a flexible reweighting technique for training deep learning models.
We conclude that the proposed method adapts the sample importance, which leverages the performance
on a challenging multisite, multiorgan, and multisize segmentation task.
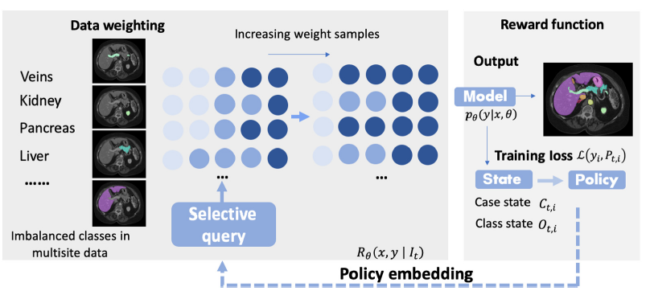