Efficient approximate signal reconstruction for correction of gradient nonlinearities in diffusion-weighted imaging
Posted by kanakap on Thursday, August 31, 2023 in Diffusion Tensor Imaging, Diffusion Weighted MRI, Image Processing, Magnetic resonance imaging, News.
Abstract
In diffusion weighted MRI (DW-MRI), hardware nonlinearities lead to spatial variations in the orientation and magnitude of diffusion weighting. While the correction of these spatial distortions has been well established for analyses of DW-MRI, the existing voxel-wise empirical correction for gradient nonlinearities requires reimplementation of existing models, as the resultant gradients vary by voxel. Herein, we propose a two-step signal approximation after voxel-wise correction of gradient nonlinearity effects in DW-MRI. The proposed technique (1) scales the diffusion signal and (2) resamples the gradient orientations. This results in uniform gradients across the corrected image and provides the key advantage of seamless integration into current diffusion workflows. We investigated the validity of our technique by fitting a multi-compartment neurite orientation dispersion and density imaging (NODDI) model to the empirical correction and proposed approximation in five subjects from the MASiVar pediatric dataset. We evaluated intra-cellular volume fraction (iVF), CSF volume fraction (cVF), and orientation dispersion index (ODI) from NODDI. The Cohen’s d of iVF, cVF and ODI between the techniques was <0.2 indicating the proposed technique does not exhibit significant differences from the voxel-wise correction technique. Our two-step signal approximation is an efficient representation of the voxel-wise gradient table correction. Using this approximation, correction of gradient nonlinearities can be easily incorporated into existing diffusion preprocessing pipelines and is implemented in “PreQual: An automated pipeline for integrated preprocessing and quality assurance of diffusion weighted MRI images”.
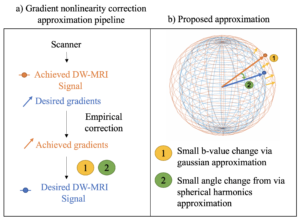