Development and Characterization of a Chest CT Atlas
Kaiwen Xu, Riqiang Gao, Mirza S. Khan, Shunxing Bao, Yucheng Tang, Steve A. Deppen, Yuankai Huo, Kim L. Sandler, Pierre P. Massion, Mattias P. Heinrich, Bennett A. Landman. Development and characterization of a chest CT atlas. SPIE Medical Imaging, 2021.
Full text: https://arxiv.org/abs/2012.03124
Abstract
A major goal of lung cancer screening is to identify individuals with particular phenotypes that are associated with high risk of cancer. Identifying relevant phenotypes is complicated by the variation in body position and body composition. In the brain, standardized coordinate systems (e.g., atlases) have enabled separate consideration of local features from gross/global structure. To date, no analogous standard atlas has been presented to enable spatial mapping and harmonization in chest computational tomography (CT). In this paper, we propose a thoracic atlas built upon a large low dose CT (LDCT) database of lung cancer screening program. The study cohort includes 466 male and 387 female subjects with no screening detected malignancy (age 46-79 years, mean 64.9 years). To provide spatial mapping, we optimize a multi-stage inter-subject non-rigid registration pipeline for the entire thoracic space. Briefly, with 50 scans of 50 randomly selected female subjects as fine tuning dataset, we search for the optimal configuration of the non-rigid registration module in a range of adjustable parameters including: registration searching radius, degree of keypoint dispersion, regularization coefficient and similarity patch size, to minimize the registration failure rate approximated by the number of samples with low Dice similarity score (DSC) for lung and body segmentation. We evaluate the optimized pipeline on a separate cohort (100 scans of 50 female and 50 male subjects) relative to two baselines with alternative non-rigid registration module: the same software with default parameters and an alternative software. We achieve a significant improvement in terms of registration success rate based on manual QA. For the entire study cohort, the optimized pipeline achieves a registration success rate of 91.7%. The application validity of the developed atlas is evaluated in terms of discriminative capability for different anatomic phenotypes, including body mass index (BMI), chronic obstructive pulmonary disease (COPD), and coronary artery calcification (CAC).
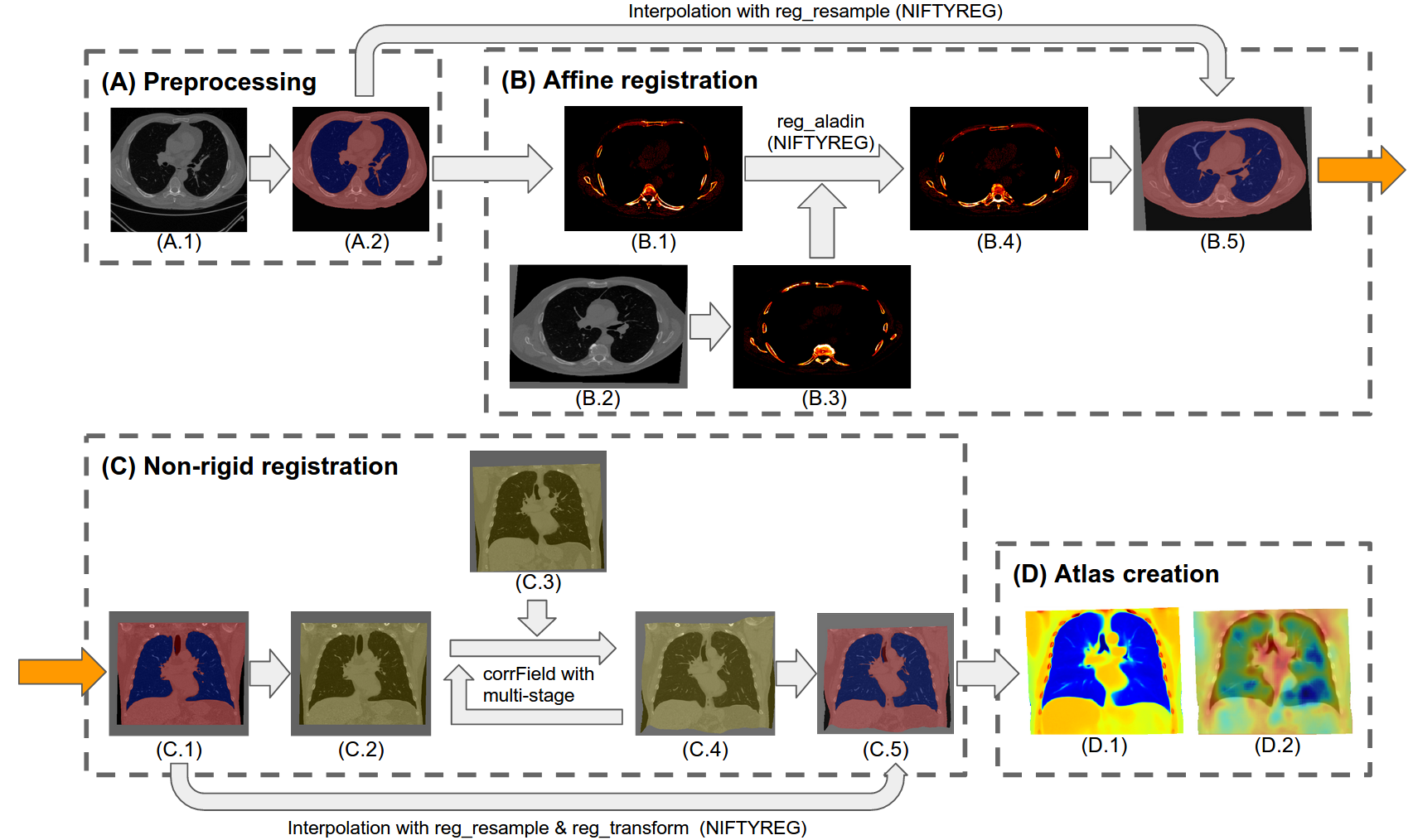