Body Part Regression With Self-Supervision
Y.Tang, R.Gao, S.Han, Y.Chen, D.Gao, V.Nath, C.Bermudez, M.R. Savona, R.G. Abramson, S.Bao,I.Lyu, Y.Huo and B.A. Landman,”Body Part Regression with Self-supervision”,IEEETransactions onMedicalImaging,2021
Full Text:
https://ieeexplore.ieee.org/stamp/stamp.jsp?arnumber=9350603
Abstract
Body part regression is a promising new technique that enables content navigation through selfsupervised learning. Using this technique, the global quantitative spatial location for each axial view slice is obtained from computed tomography (CT). However, it is challenging to define a unified global coordinate system for body CT scans due to the large variabilities in image resolution, contrasts, sequences, and patient anatomy. Therefore, the widely used supervised learning approach cannot be easily deployed. To address these concerns, we propose an annotation-free method named blind-unsupervised-supervision network (BUSN). The contributions of the work are in four folds: (1) 1030 multi-center CT scans are used in developing BUSN without any manual annotation. (2) the proposed BUSN corrects the predictions from unsupervised learning and uses the corrected results as the new supervision; (3) to improve the consistency of predictions, we propose a novel neighbor message passing (NMP) scheme that is integrated with BUSN as a statistical learning based correction; and (4) we introduce a new pre-processing pipeline with inclusion of the BUSN, which is validated on 3D multi-organ segmentation. The proposed method is trained on 1,030 whole body CT scans (230,650 slices) from five datasets,as well as an independentexternal validation cohort with 100 scans. From the body part regression results, the proposed BUSN achieved significantly higher median R squared score ( = 0.9089) than the state-ofthe-art unsupervised method (= 0.7153). When introducing BUSN as a preprocessingstage in volumetric segmentation, the proposedpre-processingpipeline using BUSN approach increases the total mean Dice score of the 3D abdominal multi-organ segmentation from 0.7991 to 0.8145.
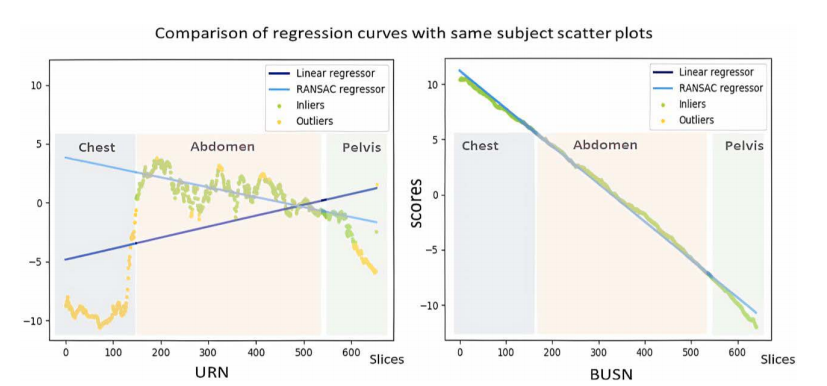