Extending the value of routine lung screening CT with quantitative body composition assessment
Kaiwen Xu, Riqiang Gao, Yucheng Tang, Steve A. Deppen, Kim L. Sandler, Michael N. Kammer, Sanja L. Antic, Fabien Maldonado, Yuankai Huo, Mirza S. Khan, Bennett A. Landman
Abstract
Certain body composition phenotypes, like sarcopenia, are well established as predictive markers for post-surgery complications and overall survival of lung cancer patients. However, their association with incidental lung cancer risk in the screening population is still unclear. We study the feasibility of body composition analysis using chest low dose computed tomography (LDCT). A two-stage fully automatic pipeline is developed to assess the cross-sectional area of body composition components including subcutaneous adipose tissue (SAT), muscle, visceral adipose tissue (VAT), and bone on T5, T8 and T10 vertebral levels. The pipeline is developed using 61 cases of the VerSe`20 dataset, 40 annotated cases of NLST, and 851 inhouse screening cases. On a test cohort consisting of 30 cases from the inhouse screening cohort (age 55 – 73, 50% female) and 42 cases of NLST (age 55 – 75, 59.5% female), the pipeline achieves a root mean square error (RMSE) of 7.25 mm (95% CI: [6.61, 7.85]) for the vertebral level identification and mean Dice similarity score (DSC) 0.99 (0.02), 0.96 (0.03), and 0.95 (0.04) for SAT, muscle, and VAT, respectively for body composition segmentation. The pipeline is generalized to the CT arm of the NLST dataset (25,205 subjects, 40.8% female, 1,056 lung cancer incidences). Time-to-event analysis for lung cancer incidence indicates inverse association between measured muscle cross-sectional area and incidental lung cancer risks (p < 0.001 female, p < 0.001 male). In conclusion, automatic body composition analysis using routine lung screening LDCT is feasible.
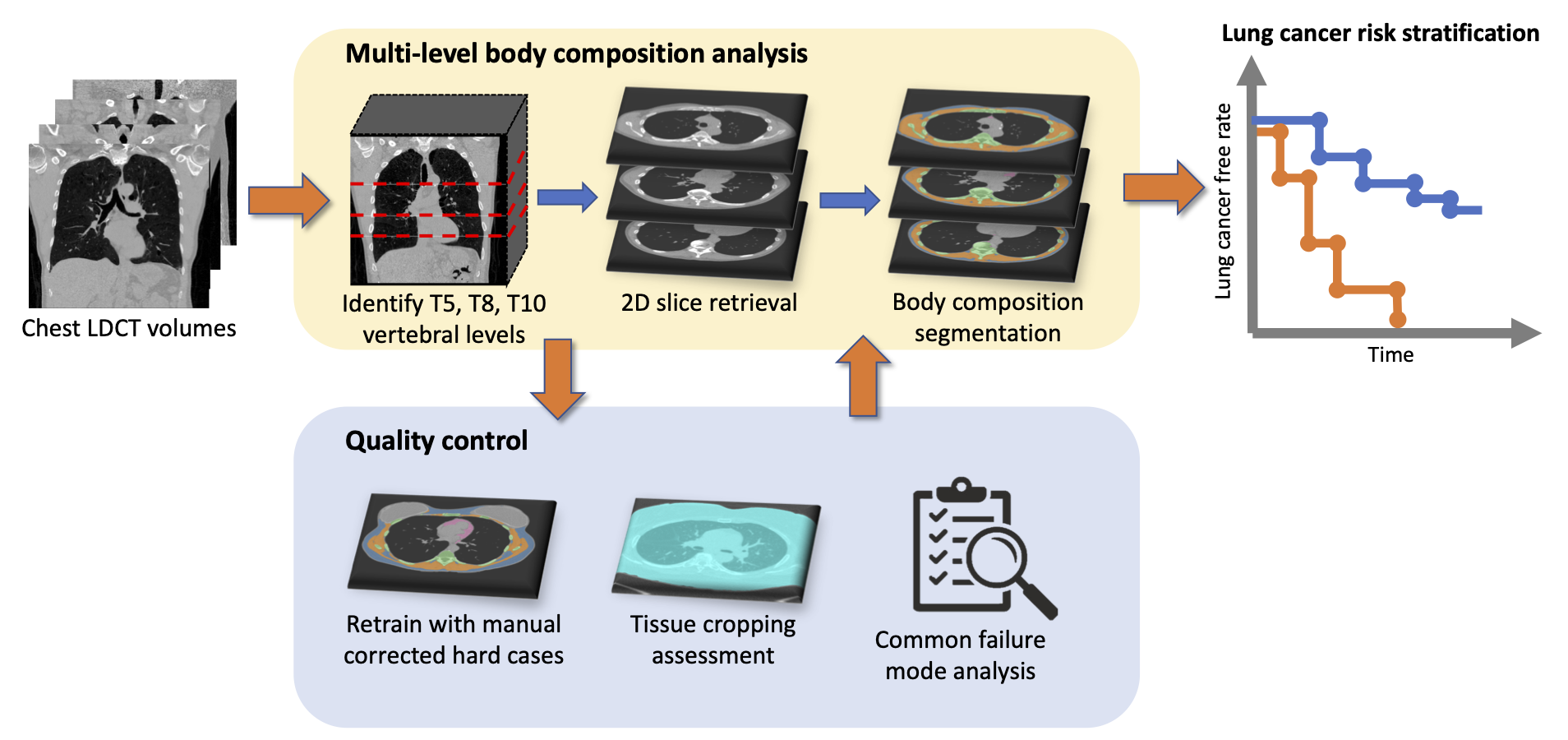